Jing Tan 谈婧
Ph.D. Student @ MMLab
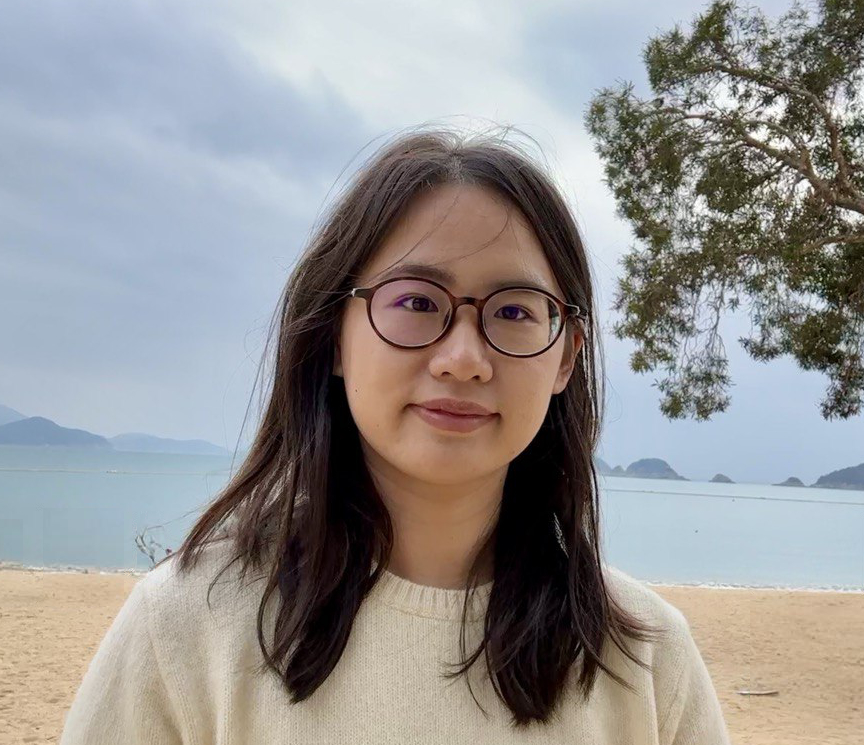
I am a 1st-year Ph.D. student in MMLab (Multimedia Laboratory), The Chinese University of Hong Kong (CUHK), supervised by Prof. Dahua Lin. I also work closely with Dr. Tong Wu at MMLab, CUHK. Before that, I obtained my Master degree from the Department of Computer Science and Technology, Nanjing University, supervised by Prof. Limin Wang. I obtained the B.Sc. degree from Kuang Yaming Honors School, Nanjing University in 2020.
My research focuses on perceiving the world from understanding the foreground events to the creation of the 3D background.
Currently, I’m working on topics including but not limited to:
- 3D Scene Creation from Limited View,
- Action Localization and Detection in Videos.
I’m open to potential collaborations, please feel free to drop me an email if interested.
News
Mar, 2024 | One paper is accepted to CVPR. |
---|---|
May, 2023 | I will start my doctoral research at MMLab CUHK in 2023 fall. |
May, 2023 | Temporal Perceiver is accepted to T-PAMI! |